Questo articolo è la terza parte della “mini-serie” che descrivere come implementare un report delle performance di una strategia in Python per verificare i risultati di un backtest. In particolare offre una panoramica su come creare un generatore HTML personalizzabile per un report di una strategia di trading.
Nel precedente articolo abbiamo descritto come produrre un report delle performance con il grafico dell’equity della strategia completo di indicatori KPI e il grafico del drawdown della strategia.
Report con indicatori statistici
Vediamo ora come aggiungere altre tabelle KPI per mostrare varie statistiche e metriche, sia per la strategia che per il benchmark. In questo momento descriviamo come creare le tabelle e inserirle nel report. In un secondo momento vediamo come formattarle e renderle visivamente accattivanti.
Sfruttiamo nuovamente la potenza della libreria FFN
. Usiamo il metodo calc_stats
per effettuare il calcolo delle statistiche ed indicatori KPI. In particolare creiamo i nuovi metodi, create_kpi_table_full
e split_kpi_table
. Il primo metodo crea una tabella KPI per la strategia e per il benchmark. il secondo metodo divide la tabella in 5 tabelle più piccole puramente per motivi estetici. In questo modo possiamo adattare la tabella e permette al contenuto della pagina di essere più “fluido”.
Come sempre dobbiamo anche modificare il metodo generate_html
per chiamare nuovi metodi e creare gli elementi HTML che dobbiamo inserire nel file template.html
. Di seguito è riportato il codice che incorpora questi aggiornamenti sia per main.py
che per template.html
.
import os
import pandas as pd
import numpy as np
import plotly
import plotly.graph_objs as go
import ffn
from jinja2 import Environment, FileSystemLoader
class PerformanceReport:
""" Report con le statistiche delle performance stats per una data strategia
"""
def __init__(self, infilename):
self.infilename = infilename
self.get_data()
def get_data(self):
basedir = os.path.abspath(os.path.dirname('__file__'))
data_folder = os.path.join(basedir, 'data')
data = pd.read_csv(os.path.join(data_folder, self.infilename), index_col='date',
parse_dates=True, dayfirst=True)
self.equity_curve = data['equity_curve']
if len(data.columns) > 1:
self.benchmark_curve = data['benchmark']
def generate_html(self):
env = Environment(loader=FileSystemLoader('.'))
template = env.get_template("templates/template.html")
perf_chart = self.plot_performance_chart()
drawdown_chart = self.plot_drawdown_chart()
monthly_table = self.create_monthly_table(self.equity_curve.pct_change().dropna(), 1)
equity_curve_ffn_stats = self.get_ffn_stats(self.equity_curve)
benchmark_curve_ffn_stats = self.get_ffn_stats(self.benchmark_curve)
kpi_table = self.create_kpi_table(equity_curve_ffn_stats)
kpi_table_full = self.create_kpi_table_full([equity_curve_ffn_stats, benchmark_curve_ffn_stats])
kpi_table_1, kpi_table_2, kpi_table_3, kpi_table_4, kpi_table_5 = self.split_kpi_table(kpi_table_full)
html_out = template.render(perf_chart=perf_chart, drawdown_chart=drawdown_chart, monthly_table=monthly_table,
kpi_table=kpi_table, kpi_table_1=kpi_table_1, kpi_table_2=kpi_table_2,
kpi_table_3=kpi_table_3, kpi_table_4=kpi_table_4, kpi_table_5=kpi_table_5)
return html_out
def generate_html_report(self):
""" Restitusice un report HTML con le analisi
"""
html = self.generate_html()
outputdir = "output"
outfile = os.path.join(outputdir, 'report.html')
file = open(outfile, "w")
file.write(html)
file.close()
def rebase_series(self, series):
return (series / series.iloc[0]) * 100
def plot_performance_chart(self):
trace_equity = go.Scatter(
x=self.equity_curve.index.tolist(),
y=self.rebase_series(self.equity_curve).values.tolist(),
name='strategy',
yaxis='y2',
line=dict(color=('rgb(22, 96, 167)')))
trace_benchmark = go.Scatter(
x=self.benchmark_curve.index.tolist(),
y=self.rebase_series(self.benchmark_curve).values.tolist(),
name='benchmark',
yaxis='y2',
line=dict(color=('rgb(22, 96, 0)')))
layout = go.Layout(
autosize=True,
legend=dict(orientation="h"),
title='Performance Chart',
yaxis=dict(
title='Performance'))
perf_chart = plotly.offline.plot({"data": [trace_equity, trace_benchmark],
"layout": layout}, include_plotlyjs=False,
output_type='div')
return (perf_chart)
def plot_drawdown_chart(self):
trace_equity_drawdown = go.Scatter(
x=self.equity_curve.to_drawdown_series().index.tolist(),
y=self.equity_curve.to_drawdown_series().values.tolist(),
name='strategy drawdown',
yaxis='y2',
line=dict(color=('rgb(22, 96, 167)')))
trace_benchmark_drawdown = go.Scatter(
x=self.benchmark_curve.to_drawdown_series().index.tolist(),
y=self.benchmark_curve.to_drawdown_series().values.tolist(),
name='benchmark drawdown',
yaxis='y2',
line=dict(color=('rgb(22, 96, 0)')))
layout = go.Layout(
autosize=True,
legend=dict(orientation="h"),
title='Drawdown Chart',
yaxis=dict(
title='Drawdown'))
drawdown_chart = plotly.offline.plot({"data": [trace_equity_drawdown, trace_benchmark_drawdown],
"layout": layout}, include_plotlyjs=False,
output_type='div')
return (drawdown_chart)
def create_monthly_table(self, return_series, num_of_compenents):
return_series.rename('weighted rets', inplace=True)
return_series = (return_series / float(num_of_compenents))
returns_df_m = pd.DataFrame((return_series + 1).resample('M').prod() - 1)
returns_df_m['Month'] = returns_df_m.index.month
monthly_table = returns_df_m[['weighted rets', 'Month']].pivot_table(returns_df_m[['weighted rets', 'Month']],
index=returns_df_m.index, columns='Month',
aggfunc=np.sum).resample('A')
monthly_table = monthly_table.aggregate('sum')
monthly_table.columns = monthly_table.columns.droplevel()
monthly_table.index = monthly_table.index.year
monthly_table['YTD'] = ((monthly_table + 1).prod(axis=1) - 1)
monthly_table = monthly_table * 100
monthly_table.columns = ['Jan', 'Feb', 'Mar', 'Apr', 'May', 'Jun', 'Jul', 'Aug', 'Sep', 'Oct', 'Nov', 'Dec',
'YTD']
return monthly_table.round(2).fillna("").to_html(classes="table table-hover table-bordered table-striped")
def get_ffn_stats(self, equity_series):
equity_stats = equity_series.calc_stats()
d = dict(equity_stats.stats)
return d
def create_kpi_table(self, ffn_dict):
kpi_table = pd.DataFrame.from_dict(ffn_dict, orient='index')
kpi_table.index.name = 'KPI'
kpi_table.columns = ['Value']
kpi_table2 = kpi_table.loc[['total_return', 'cagr', 'daily_sharpe',
'daily_vol', 'max_drawdown', 'avg_drawdown']] # .astype(float)
kpi_table2['Value'] = pd.Series(["{0:.2f}%".format(val * 100) for val in kpi_table2['Value']],
index=kpi_table2.index)
kpi_table2.loc['avg_drawdown_days'] = kpi_table.loc['avg_drawdown_days']
return kpi_table2.to_html(classes="table table-hover table-bordered table-striped", header=False)
def create_kpi_table_full(self, ffn_dict_list):
df_list = [pd.DataFrame.from_dict(x, orient='index') for x in ffn_dict_list]
kpi_table_full = pd.concat(df_list, axis=1)
return kpi_table_full
def split_kpi_table(self, kpi_table_full):
kpi_table_1 = kpi_table_full.iloc[3:16].to_html(classes="table table-hover table-bordered table-striped",
header=False)
kpi_table_2 = kpi_table_full.iloc[16:24].to_html(classes="table table-hover table-bordered table-striped",
header=False)
kpi_table_3 = kpi_table_full.iloc[24:32].to_html(classes="table table-hover table-bordered table-striped",
header=False)
kpi_table_4 = kpi_table_full.iloc[32:40].to_html(classes="table table-hover table-bordered table-striped",
header=False)
kpi_table_5 = kpi_table_full.iloc[40:].to_html(classes="table table-hover table-bordered table-striped",
header=False)
return kpi_table_1, kpi_table_2, kpi_table_3, kpi_table_4, kpi_table_5
if __name__ == "__main__":
report = PerformanceReport('data.csv')
report.generate_html_report()
App
Trading Backtest Report
{{ perf_chart|safe }}
KPI Table
{{ kpi_table|safe }}
Monthly Returns
{{ monthly_table|safe }}
{{ drawdown_chart|safe }}
KPI Tables
{{ kpi_table_1|safe }}
{{ kpi_table_5|safe }}
{{ kpi_table_2|safe }}
{{ kpi_table_3|safe }}
{{ kpi_table_4|safe }}
Dopo aver eseguito lo script otteniamo il seguente report.
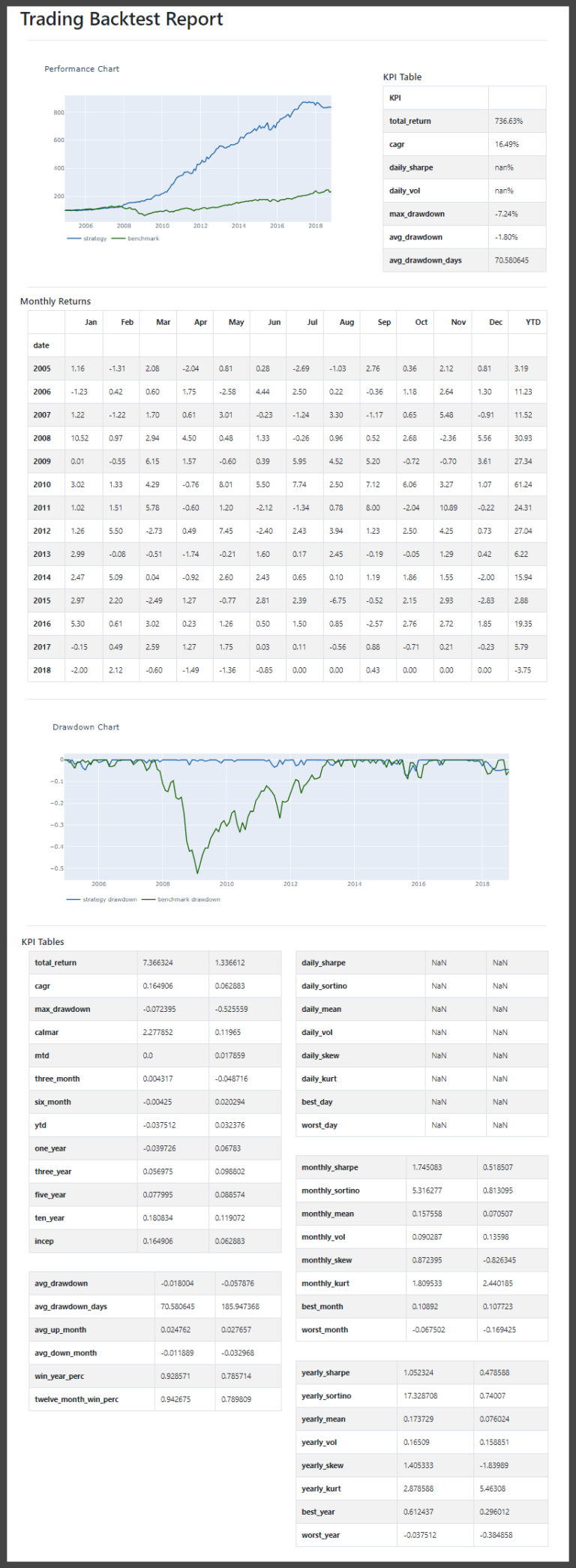
Report con istogramma dei rendimenti
Aggiungiamo un istogramma per mostrare la distribuzione dei rendimenti giornalieri, sia per la nostra strategia che per il benchmark. Prevediamo di sovrapporre i due istogrammi uno sopra l’altro e tracciarli sullo stesso asse. In questo modo possiamo fare più facilmente un confronto diretto. Per rendere il confronto dobbiamo assicurarci che le dimensioni dei contenitori siano le stesse per entrambe le serie.
A tale scopo dobbiamo aggiungere il metodo plot_daily_histogram
alla classe PerformanceReport
come riportato nel codice seguente. Il metodo crea l’oggetto HTML necessario per inserire l’istogramma nel modello HTML. Come sempre, sono state apportate modifiche al metodo generate_html
per poter chiamare il nuovo metodo plot_daily_histogram
e passarlo al modello HTML.
Nel file “template.html” abbiamo aggiunto una nuova riga e una colonna div contenente la variabile inserita.
Di seguito riportiamo i file aggiornati.
import os
import pandas as pd
import numpy as np
import plotly
import plotly.graph_objs as go
import ffn
from jinja2 import Environment, FileSystemLoader
class PerformanceReport:
""" Report con le statistiche delle performance stats per una data strategia
"""
def __init__(self, infilename):
self.infilename = infilename
self.get_data()
def get_data(self):
basedir = os.path.abspath(os.path.dirname('__file__'))
data_folder = os.path.join(basedir, 'data')
data = pd.read_csv(os.path.join(data_folder, self.infilename), index_col='date',
parse_dates=True, dayfirst=True)
self.equity_curve = data['equity_curve']
if len(data.columns) > 1:
self.benchmark_curve = data['benchmark']
def generate_html(self):
env = Environment(loader=FileSystemLoader('.'))
template = env.get_template("templates/template.html")
perf_chart = self.plot_performance_chart()
drawdown_chart = self.plot_drawdown_chart()
monthly_table = self.create_monthly_table(self.equity_curve.pct_change().dropna(), 1)
equity_curve_ffn_stats = self.get_ffn_stats(self.equity_curve)
benchmark_curve_ffn_stats = self.get_ffn_stats(self.benchmark_curve)
kpi_table = self.create_kpi_table(equity_curve_ffn_stats)
kpi_table_full = self.create_kpi_table_full([equity_curve_ffn_stats, benchmark_curve_ffn_stats])
kpi_table_1, kpi_table_2, kpi_table_3, kpi_table_4, kpi_table_5 = self.split_kpi_table(kpi_table_full)
daily_ret_hist = self.plot_daily_histogram()
html_out = template.render(perf_chart=perf_chart, drawdown_chart=drawdown_chart, monthly_table=monthly_table,
kpi_table=kpi_table, kpi_table_1=kpi_table_1, kpi_table_2=kpi_table_2,
kpi_table_3=kpi_table_3, kpi_table_4=kpi_table_4, kpi_table_5=kpi_table_5,
daily_ret_hist=daily_ret_hist)
return html_out
def generate_html_report(self):
""" Restitusice un report HTML con le analisi
"""
html = self.generate_html()
outputdir = "output"
outfile = os.path.join(outputdir, 'report.html')
file = open(outfile, "w")
file.write(html)
file.close()
def rebase_series(self, series):
return (series / series.iloc[0]) * 100
def plot_performance_chart(self):
trace_equity = go.Scatter(
x=self.equity_curve.index.tolist(),
y=self.rebase_series(self.equity_curve).values.tolist(),
name='strategy',
yaxis='y2',
line=dict(color=('rgb(22, 96, 167)')))
trace_benchmark = go.Scatter(
x=self.benchmark_curve.index.tolist(),
y=self.rebase_series(self.benchmark_curve).values.tolist(),
name='benchmark',
yaxis='y2',
line=dict(color=('rgb(22, 96, 0)')))
layout = go.Layout(
autosize=True,
legend=dict(orientation="h"),
title='Performance Chart',
yaxis=dict(
title='Performance'))
perf_chart = plotly.offline.plot({"data": [trace_equity, trace_benchmark],
"layout": layout}, include_plotlyjs=False,
output_type='div')
return (perf_chart)
def plot_drawdown_chart(self):
trace_equity_drawdown = go.Scatter(
x=self.equity_curve.to_drawdown_series().index.tolist(),
y=self.equity_curve.to_drawdown_series().values.tolist(),
name='strategy drawdown',
yaxis='y2',
line=dict(color=('rgb(22, 96, 167)')))
trace_benchmark_drawdown = go.Scatter(
x=self.benchmark_curve.to_drawdown_series().index.tolist(),
y=self.benchmark_curve.to_drawdown_series().values.tolist(),
name='benchmark drawdown',
yaxis='y2',
line=dict(color=('rgb(22, 96, 0)')))
layout = go.Layout(
autosize=True,
legend=dict(orientation="h"),
title='Drawdown Chart',
yaxis=dict(
title='Drawdown'))
drawdown_chart = plotly.offline.plot({"data": [trace_equity_drawdown, trace_benchmark_drawdown],
"layout": layout}, include_plotlyjs=False,
output_type='div')
return (drawdown_chart)
def create_monthly_table(self, return_series, num_of_compenents):
return_series.rename('weighted rets', inplace=True)
return_series = (return_series / float(num_of_compenents))
returns_df_m = pd.DataFrame((return_series + 1).resample('M').prod() - 1)
returns_df_m['Month'] = returns_df_m.index.month
monthly_table = returns_df_m[['weighted rets', 'Month']].pivot_table(returns_df_m[['weighted rets', 'Month']],
index=returns_df_m.index, columns='Month',
aggfunc=np.sum).resample('A')
monthly_table = monthly_table.aggregate('sum')
monthly_table.columns = monthly_table.columns.droplevel()
monthly_table.index = monthly_table.index.year
monthly_table['YTD'] = ((monthly_table + 1).prod(axis=1) - 1)
monthly_table = monthly_table * 100
monthly_table.columns = ['Jan', 'Feb', 'Mar', 'Apr', 'May', 'Jun', 'Jul', 'Aug', 'Sep', 'Oct', 'Nov', 'Dec',
'YTD']
return monthly_table.round(2).fillna("").to_html(classes="table table-hover table-bordered table-striped")
def get_ffn_stats(self, equity_series):
equity_stats = equity_series.calc_stats()
d = dict(equity_stats.stats)
return d
def create_kpi_table(self, ffn_dict):
kpi_table = pd.DataFrame.from_dict(ffn_dict, orient='index')
kpi_table.index.name = 'KPI'
kpi_table.columns = ['Value']
kpi_table2 = kpi_table.loc[['total_return', 'cagr', 'daily_sharpe',
'daily_vol', 'max_drawdown', 'avg_drawdown']] # .astype(float)
kpi_table2['Value'] = pd.Series(["{0:.2f}%".format(val * 100) for val in kpi_table2['Value']],
index=kpi_table2.index)
kpi_table2.loc['avg_drawdown_days'] = kpi_table.loc['avg_drawdown_days']
return kpi_table2.to_html(classes="table table-hover table-bordered table-striped", header=False)
def create_kpi_table_full(self, ffn_dict_list):
df_list = [pd.DataFrame.from_dict(x, orient='index') for x in ffn_dict_list]
kpi_table_full = pd.concat(df_list, axis=1)
return kpi_table_full
def split_kpi_table(self, kpi_table_full):
kpi_table_1 = kpi_table_full.iloc[3:16].to_html(classes="table table-hover table-bordered table-striped",
header=False)
kpi_table_2 = kpi_table_full.iloc[16:24].to_html(classes="table table-hover table-bordered table-striped",
header=False)
kpi_table_3 = kpi_table_full.iloc[24:32].to_html(classes="table table-hover table-bordered table-striped",
header=False)
kpi_table_4 = kpi_table_full.iloc[32:40].to_html(classes="table table-hover table-bordered table-striped",
header=False)
kpi_table_5 = kpi_table_full.iloc[40:].to_html(classes="table table-hover table-bordered table-striped",
header=False)
return kpi_table_1, kpi_table_2, kpi_table_3, kpi_table_4, kpi_table_5
def plot_daily_histogram(self):
trace0 = go.Histogram(x=self.equity_curve.pct_change().values,
name="Strategy",
opacity=0.75,
marker=dict(
color=('rgb(22, 96, 167)')),
xbins=dict(
size=0.0025
))
trace1 = go.Histogram(x=self.benchmark_curve.pct_change().values,
name="Benchmark",
opacity=0.75,
marker=dict(
color=('rgb(22, 96, 0)')),
xbins=dict(
size=0.0025
))
data = [trace0, trace1]
layout = go.Layout(
title='Histogram of Strategy and Benchmark Daily Returns',
autosize=True,
height=600,
hovermode='closest',
barmode='overlay'
)
daily_ret_hist = plotly.offline.plot({"data": data, "layout": layout},
include_plotlyjs=False,
output_type='div')
return daily_ret_hist
if __name__ == "__main__":
report = PerformanceReport('data.csv')
report.generate_html_report()
App
Trading Backtest Report
{{ perf_chart|safe }}
KPI Table
{{ kpi_table|safe }}
Monthly Returns
{{ monthly_table|safe }}
{{ drawdown_chart|safe }}
KPI Tables
{{ kpi_table_1|safe }}
{{ kpi_table_5|safe }}
{{ kpi_table_2|safe }}
{{ kpi_table_3|safe }}
{{ kpi_table_4|safe }}
{{ daily_ret_hist|safe }}
Il report ottenuto ha questo aspetto. Per sintesi mostriamo solamente l’ultima parte e non i grafici di equity e drawdown che non hanno subito modifiche.
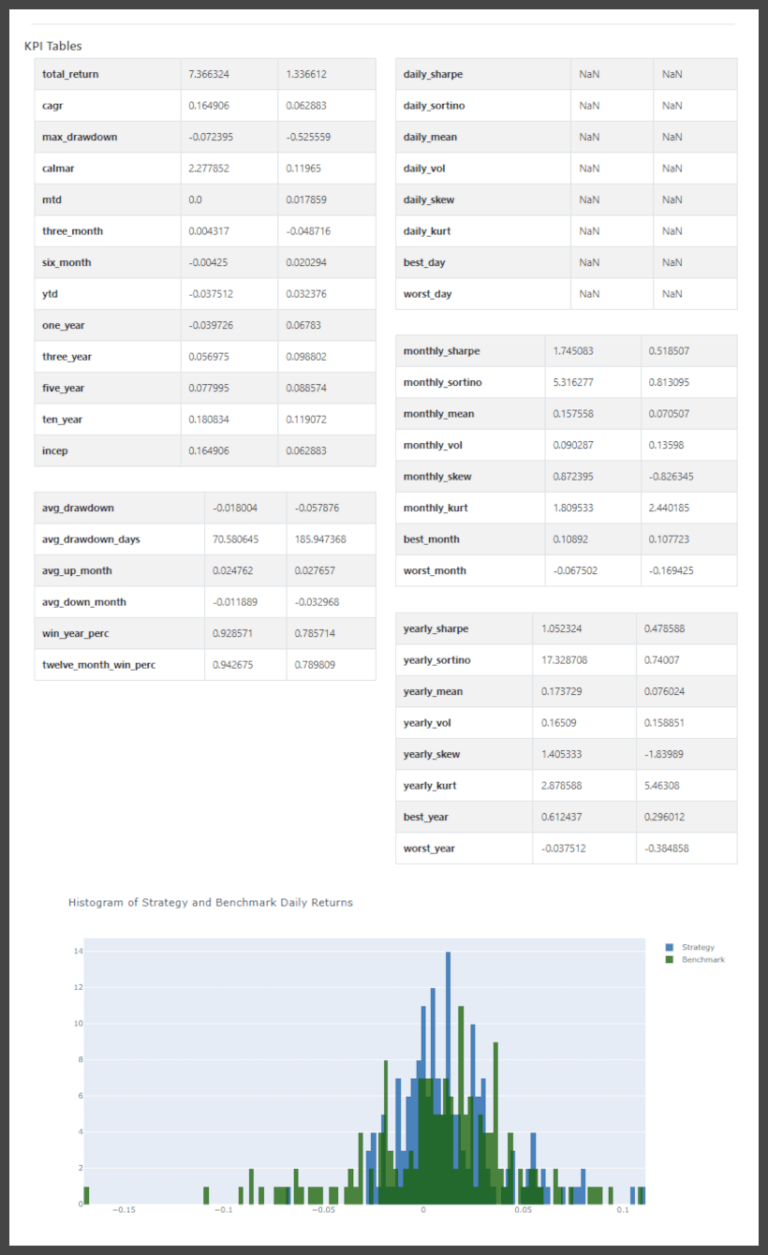
Report con boxplot
Molti analisti pensano che i boxplot siano molto utili, insieme agli istogrammi, durante l’analisi delle distribuzioni. Aggiungiamo quindi al report un boxplot dei rendimenti giornalieri per la strategia e per il benchmark. Dobbiamo aggiungere il nuovo metodo plot_daily_box
al file main.py
e modificare opportunamente il metodo generate_html
.
Dopo aver implementato le modifiche ed apportano alcune piccole migliorie estetiche, come la ridenominazione dei titoli dei grafici, il file main.py
ha questo aspetto.
import os
import pandas as pd
import numpy as np
import plotly
import plotly.graph_objs as go
import ffn
from jinja2 import Environment, FileSystemLoader
class PerformanceReport:
""" Report con le statistiche delle performance stats per una data strategia
"""
def __init__(self, infilename):
self.infilename = infilename
self.get_data()
def get_data(self):
basedir = os.path.abspath(os.path.dirname('__file__'))
data_folder = os.path.join(basedir, 'data')
data = pd.read_csv(os.path.join(data_folder, self.infilename), index_col='date',
parse_dates=True, dayfirst=True)
self.equity_curve = data['equity_curve']
if len(data.columns) > 1:
self.benchmark_curve = data['benchmark']
def generate_html(self):
env = Environment(loader=FileSystemLoader('.'))
template = env.get_template("templates/template.html")
perf_chart = self.plot_performance_chart()
drawdown_chart = self.plot_drawdown_chart()
monthly_table = self.create_monthly_table(self.equity_curve.pct_change().dropna(), 1)
equity_curve_ffn_stats = self.get_ffn_stats(self.equity_curve)
benchmark_curve_ffn_stats = self.get_ffn_stats(self.benchmark_curve)
kpi_table = self.create_kpi_table(equity_curve_ffn_stats)
kpi_table_full = self.create_kpi_table_full([equity_curve_ffn_stats, benchmark_curve_ffn_stats])
kpi_table_1, kpi_table_2, kpi_table_3, kpi_table_4, kpi_table_5 = self.split_kpi_table(kpi_table_full)
daily_ret_hist = self.plot_daily_histogram()
daily_ret_box = self.plot_daily_box()
html_out = template.render(perf_chart=perf_chart, drawdown_chart=drawdown_chart, monthly_table=monthly_table,
kpi_table=kpi_table, kpi_table_1=kpi_table_1, kpi_table_2=kpi_table_2,
kpi_table_3=kpi_table_3, kpi_table_4=kpi_table_4, kpi_table_5=kpi_table_5,
daily_ret_hist=daily_ret_hist, daily_ret_box=daily_ret_box)
return html_out
def generate_html_report(self):
""" Restitusice un report HTML con le analisi
"""
html = self.generate_html()
outputdir = "output"
outfile = os.path.join(outputdir, 'report.html')
file = open(outfile, "w")
file.write(html)
file.close()
def rebase_series(self, series):
return (series / series.iloc[0]) * 100
def plot_performance_chart(self):
trace_equity = go.Scatter(
x=self.equity_curve.index.tolist(),
y=self.rebase_series(self.equity_curve).values.tolist(),
name='Strategy',
yaxis='y2',
line=dict(color=('rgb(22, 96, 167)')))
trace_benchmark = go.Scatter(
x=self.benchmark_curve.index.tolist(),
y=self.rebase_series(self.benchmark_curve).values.tolist(),
name='Benchmark',
yaxis='y2',
line=dict(color=('rgb(22, 96, 0)')))
layout = go.Layout(
autosize=True,
legend=dict(orientation="h"),
title='Performance Chart',
yaxis=dict(
title='Performance'))
perf_chart = plotly.offline.plot({"data": [trace_equity, trace_benchmark],
"layout": layout}, include_plotlyjs=False,
output_type='div')
return perf_chart
def plot_drawdown_chart(self):
trace_equity_drawdown = go.Scatter(
x=self.equity_curve.to_drawdown_series().index.tolist(),
y=self.equity_curve.to_drawdown_series().values.tolist(),
name='Strategy',
yaxis='y2',
line=dict(color=('rgb(22, 96, 167)')))
trace_benchmark_drawdown = go.Scatter(
x=self.benchmark_curve.to_drawdown_series().index.tolist(),
y=self.benchmark_curve.to_drawdown_series().values.tolist(),
name='Benchmark',
yaxis='y2',
line=dict(color=('rgb(22, 96, 0)')))
layout = go.Layout(
autosize=True,
legend=dict(orientation="h"),
title='Drawdown Chart',
yaxis=dict(
title='Drawdown'))
drawdown_chart = plotly.offline.plot({"data": [trace_equity_drawdown, trace_benchmark_drawdown],
"layout": layout}, include_plotlyjs=False,
output_type='div')
return drawdown_chart
def create_monthly_table(self, return_series, num_of_compenents):
return_series.rename('weighted rets', inplace=True)
return_series = (return_series / float(num_of_compenents))
returns_df_m = pd.DataFrame((return_series + 1).resample('M').prod() - 1)
returns_df_m['Month'] = returns_df_m.index.month
monthly_table = returns_df_m[['weighted rets', 'Month']].pivot_table(returns_df_m[['weighted rets', 'Month']],
index=returns_df_m.index, columns='Month',
aggfunc=np.sum).resample('A')
monthly_table = monthly_table.aggregate('sum')
monthly_table.columns = monthly_table.columns.droplevel()
monthly_table.index = monthly_table.index.year
monthly_table['YTD'] = ((monthly_table + 1).prod(axis=1) - 1)
monthly_table = monthly_table * 100
monthly_table.columns = ['Jan', 'Feb', 'Mar', 'Apr', 'May', 'Jun', 'Jul', 'Aug', 'Sep', 'Oct', 'Nov', 'Dec',
'YTD']
return monthly_table.round(2).fillna("").to_html(classes="table table-hover table-bordered table-striped")
def get_ffn_stats(self, equity_series):
equity_stats = equity_series.calc_stats()
d = dict(equity_stats.stats)
return d
def create_kpi_table(self, ffn_dict):
kpi_table = pd.DataFrame.from_dict(ffn_dict, orient='index')
kpi_table.index.name = 'KPI'
kpi_table.columns = ['Value']
kpi_table2 = kpi_table.loc[['total_return', 'cagr',
'daily_vol', 'max_drawdown', 'avg_drawdown']] # .astype(float)
kpi_table2['Value'] = pd.Series(["{0:.2f}%".format(val * 100) for val in kpi_table2['Value']],
index=kpi_table2.index)
kpi_table2.loc['avg_drawdown_days'] = kpi_table.loc['avg_drawdown_days']
kpi_table2.loc['daily_sharpe'] = np.round(kpi_table.loc['daily_sharpe'].values[0], 2)
return kpi_table2.to_html(classes="table table-hover table-bordered table-striped", header=False)
def create_kpi_table_full(self, ffn_dict_list):
df_list = [pd.DataFrame.from_dict(x, orient='index') for x in ffn_dict_list]
kpi_table_full = pd.concat(df_list, axis=1)
return kpi_table_full
def split_kpi_table(self, kpi_table_full):
kpi_table_1 = kpi_table_full.iloc[3:16].to_html(classes="table table-hover table-bordered table-striped",
header=False)
kpi_table_2 = kpi_table_full.iloc[16:24].to_html(classes="table table-hover table-bordered table-striped",
header=False)
kpi_table_3 = kpi_table_full.iloc[24:32].to_html(classes="table table-hover table-bordered table-striped",
header=False)
kpi_table_4 = kpi_table_full.iloc[32:40].to_html(classes="table table-hover table-bordered table-striped",
header=False)
kpi_table_5 = kpi_table_full.iloc[40:].to_html(classes="table table-hover table-bordered table-striped",
header=False)
return kpi_table_1, kpi_table_2, kpi_table_3, kpi_table_4, kpi_table_5
def plot_daily_histogram(self):
trace0 = go.Histogram(x=self.equity_curve.pct_change().values,
name="Strategy",
opacity=0.75,
marker=dict(
color=('rgb(22, 96, 167)')),
xbins=dict(
size=0.0025
))
trace1 = go.Histogram(x=self.benchmark_curve.pct_change().values,
name="Benchmark",
opacity=0.75,
marker=dict(
color=('rgb(22, 96, 0)')),
xbins=dict(
size=0.0025
))
data = [trace0, trace1]
layout = go.Layout(
title='Histogram of Strategy and Benchmark Daily Returns',
autosize=True,
height=600,
hovermode='closest',
barmode='overlay'
)
daily_ret_hist = plotly.offline.plot({"data": data, "layout": layout},
include_plotlyjs=False,
output_type='div')
return daily_ret_hist
def plot_daily_box(self):
trace0 = go.Box(y=self.equity_curve.pct_change().values,
name="Strategy",
marker=dict(
color=('rgb(22, 96, 167)')))
trace1 = go.Box(y=self.benchmark_curve.pct_change().values,
name="Benchmark",
marker=dict(
color=('rgb(22, 96, 0)')))
data = [trace0, trace1]
layout = go.Layout(
title='Boxplot of Strategy and Benchmark Daily Returns',
autosize=True,
height=600,
yaxis=dict(
zeroline=False
)
)
box_plot = plotly.offline.plot({"data": data, "layout": layout}, include_plotlyjs=False,
output_type='div')
return box_plot
if __name__ == "__main__":
report = PerformanceReport('data.csv')
report.generate_html_report()
template.html
ha il seguente aspetto.
App
Trading Backtest Report
{{ perf_chart|safe }}
KPI Table
{{ kpi_table|safe }}
Monthly Returns
{{ monthly_table|safe }}
{{ drawdown_chart|safe }}
KPI Tables
{{ kpi_table_1|safe }}
{{ kpi_table_5|safe }}
{{ kpi_table_2|safe }}
{{ kpi_table_3|safe }}
{{ kpi_table_4|safe }}
{{ daily_ret_hist|safe }}
{{ daily_ret_box|safe }}
Vediamo che il nuovo report contiene il boxplot, come mostrato di seguito.
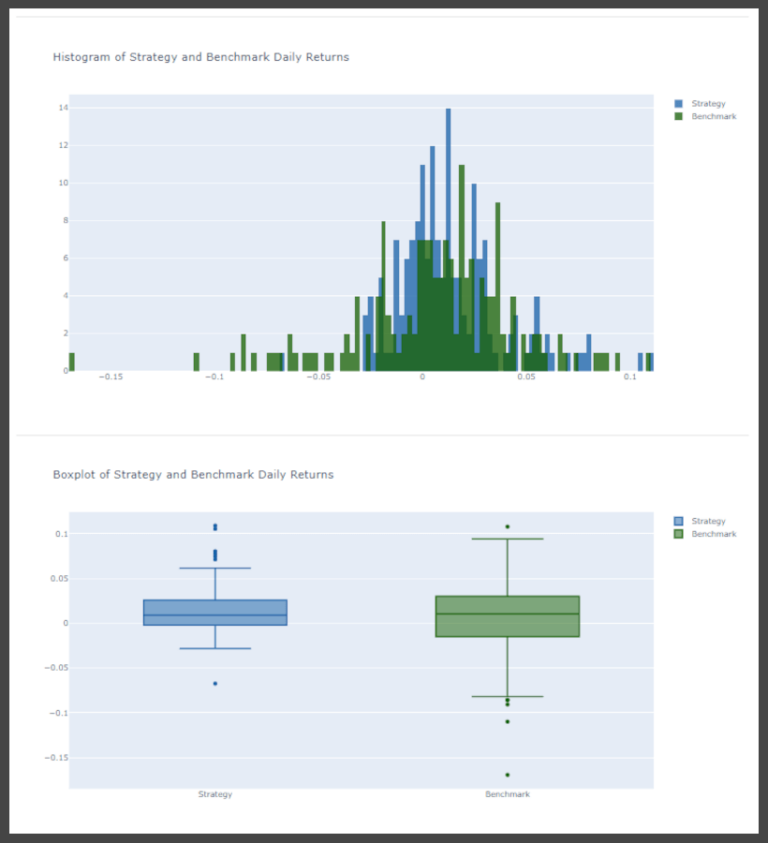
Abbiamo ottenuto un buon punto di partenza. Abbiamo aggiunto al report alcune tabelle di statistiche, insieme a un istogramma e un boxplot dei rendimenti giornalieri per la strategia e per il benchmark.
Conclusioni
Abbiamo descritto il processo con cui aggiungiamo funzionalità e metodi alla classe PerformanceReport
per restituire un oggetto con le informazioni/grafico desiderati. Queste informazioni sono convertite in HTML (se non sono in formato HTML) e iniettate nel template del report tramite gli argomenti del metodo template.render
.
Praticamente qualsiasi oggetto Python può essere inserito nel template, quindi abbiamo moltissime possibilità di personalizzazione del report. Potete usare questo progetto ed aggiungere le statistiche che ritenete più utili.
Nel prossimo articolo descriviamo come aggiungere funzionalità complesse ma molti utili per capire il processo di generazione dei report. In particolare vediamo come incorporare le simulazioni Monte Carlo per permetterci di calcolare il Value at Risk (VaR) delle strategie ed analizzare i migliori/peggiori scenari e le stime di possibili futuri drawdown. Descrivamo come usare diversi metodi per generare le simulazioni, cioè la simulazione Monte Carlo che genera possibili risultati campionando da una distribuzione teorica con parametri predefiniti e il “ricampionamento con sostituzione”, noto anche come “bootstrapping”.
Codice completo
In questo articolo abbiamo descritto come implementare un report delle performance di una strategia in Python. Per il codice completo riportato in questo articolo, si può consultare il seguente repository di github:
https://github.com/datatrading-info/AnalisiDatiFinanziari